Over the years, the topic of Artificial Intelligence has been the subject of much discussion and controversy. Today, Artificial Intelligence (AI) is beginning to impact many aspects of our daily lives and could have a major impact on future innovation and evolution.
The European Commission defines AI as: “Artificial intelligence (AI) refers to systems that display intelligent behaviour by analysing their environment and taking actions – with some degree of autonomy – to achieve specific goals. AI-based systems can be purely software-based, acting in the virtual world (e.g. voice assistants, image analysis software, search engines, speech and face recognition systems) or AI can be embedded in hardware devices (e.g., advanced robots, autonomous cars, drones or Internet of Things applications).”
In the context of HPC, AI is an intelligent software system that exhibits advanced and adaptable behaviours. The concept has existed for some time and is now being embraced rapidly and widely within research and by most sectors of industry. The term itself combines of multiple techniques to analyse data such as:
- machine learning, a process by which computers learn patterns from data and use those patterns to make predictions or decisions without being explicitly programmed for each task, with methods like deep learning, supervised learning, unsupervised learning, reinforcement learning;
- natural language processing with tools like transformers, generative pre-trained transformers (GPT) etc;
- machine perception, which refers to the ability of a machine to understand and interact with the environment which surrounds it (e.g., Using cameras, sensors, etc);
- computer vision, which enables computers to interpret and understand visual information from digital images;
- and much more…
Providing access to EuroHPC Supercomputer to resolve AI challenges:
Whether AI is used for search engines, image processing or language analyses, it always relies on computers. The larger the data or the problem it tackles, the more compute nodes are needed to handle it in a fast and accurate manner.
Since 2020, the use of high-performance computing (HPC) for AI has increased exponentially in Europe. Originally, AI in HPC was used to tackle problems within the domain of social sciences to find trends using data analysis. Today, AI in HPC is used across many scientific and non-scientific disciplines to interpret large datasets.
The first EuroHPC JU supercomputers came online and were made available to researchers in April 2021. Currently, there are six EuroHPC supercomputers across Europe which are operational and available for use: they are: Leonardo in Italy, LUMI in Finland, MeluXina in Luxembourg, Karolina in Czech Republic, Vega in Slovenia and Discoverer in Bulgaria.
Since 2021, the EuroHPC JU has received over 700 applications from researchers across Europe to use these supercomputers in different scientific disciplines or industrial sectors. More information on how to gain access can be found here.
Of these, 120 applications are related to AI, with 42 dedicated to large language model (LLM) problems, for example using LLMs to sort and analyse large amounts of text-based documents. In the table below, we can see the distribution of active AI projects across different EuroHPC JU systems. The table also indicates the different access modes, based on compute resource requirements, that applicants have requested.
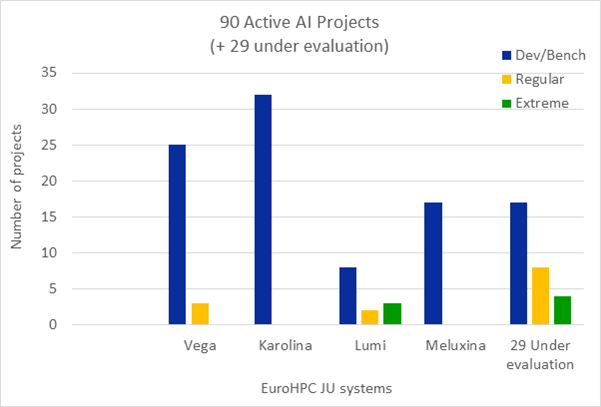
Supporting the development of AI expertise in HPC
Using supercomputers to solve AI problems is complex, and in many cases expert support is needed to ensure that these challenging AI puzzles are solved quickly and efficiently. To finance this type of expertise and support across Europe, EuroHPC JU established the EuroCC National Competence Centres (NCCs) in each country across the European Union.
AI experts from the 33 EuroCC NCCs have supported over 42 projects across the EU. In addition, NCCs regularly organise a range of training sessions related to AI techniques and tools within HPC, available for anyone to attend for free.
The EuroHPC JU has also financed a project called FF4EuroHPC, which fosters the uptake of HPC by small and medium enterprises (SMEs) across Europe. To date, 42 SMEs have been financed to conduct research and innovation experiments using HPC resources. Among these, 19 SME experiments successfully utilised AI on HPC to produce innovative solutions to complex problems within different industrial sectors:
- In one project involving chicken farming, AI and machine learning were employed to automate the labour-intensive task of monitoring chickens on large farms. Computer vision sensors were developed to count chickens, track their health, and detect issues like disease outbreaks, reducing costs and improving staff productivity. HPC was then used to train and calibrate these models, which was not feasible to do on standard personal computers, and this led to the development of a novel precision agriculture sensor combining cameras, edge computing, and IoT, enhancing poultry farming. HPC sped up model development significantly, resulting in over 90% accuracy for chicken detection and around a 10% reduction of costs and chicken mortality rates.
- Another project focused on enhancing maintenance in nautical and shipping machinery. Rising costs, breakdowns, and part shortages cause inefficiencies in this area. To address this, a Vessel Predictive Maintenance System was created, integrating voice command recognition to aid crew in predictive maintenance. However, noisy environments posed challenges, causing recognition errors. Traditional headsets were costly and could compromise crew’s environmental awareness, leading to injuries. Using AI and HPC, an SME developed a Natural Language Processing system tailored to the industry's most frequent commands, utilising noise filtering based on Deep Learning techniques for to ensure accuracy even in noisy environments. After HPC training, the algorithm achieved 95% accuracy in high noise conditions, and as a result, had the potential to cut maritime maintenance costs by up to 30%.
- A further project analysed paediatric medical imaging examinations using ionising irradiation. Calculating radiation doses in nuclear imaging such as PET posed a significant challenge for the medical and scientific community due to the lack of personalised dosimetry solutions. This is especially true in paediatric medicine, due to children's increased sensitivity to radiation and the lack of precision afforded by existing methods. A software was created to measure radiation for medical imaging, especially for children, allowing clinicians to assess internal dosimetry on an individual basis. Leveraging this solution for personalised dosimetry can reduce administered doses and mitigate the detrimental impact of radiation for a substantial number of treated children. Furthermore, this solution holds potential for extending its methodology to cater to other patient demographics.
Examples of AI projects that successfully used EuroHPC JU systems
Historians who study the early Middle Ages use the clues and information provided in ancient burial sites to gain more knowledge and understanding about the period. Glass beads are among the most common grave goods in the early Middle Ages, and their number can be estimated in the millions. The colour, size, shape, production technique and decoration of the beads that are discovered in burial sites contain much information that is relevant for historians regarding social customs, trade routes and production networks.
A research group from the University of Vienna sought to improve and validate the accuracy of existing deep embedded non-redundant clustering methods to find different informative ways to categorise the glass beads. Unfortunately, the relevant features for clustering were not known ahead of time, which is why an automatic learning approach for clustering data was sought to analyse the different beads. By using deep clustering methods combined with deep learning algorithms, the supercomputer was able to learn how to cluster automatically relevant features of the beads. In addition, these methods have been extended for non-redundant clusters of beads.
For this purpose, EuroHPC JU provided the University of Vienna with access to the GPU partition of the MeluXina system in Luxembourg, and the findings will be published later this year.
A research group from the University of Gothenburg wanted to better understand the distribution of global poverty historically and geographically. It is generally assumed that approximately 900 million people globally—one-third in Africa and another third in India—live in extreme poverty. Major global donors have deployed a stream of development programmes to break these traps. Despite the scale of the programmes, scholars have little knowledge about geographical and historical trends in global poverty distribution. To bridge these knowledge gaps, scholars must first tackle a data challenge: the scarcity of historical and geographical poverty data. The research group aims to recreate historical and geographical human-development trajectories from satellite images from 1984 to 2022, measuring poverty at unprecedented temporal and spatial detail.
To do this, they set out to train deep-learning models to predict health and living conditions using satellite images. The research group used TensorFlow on the EuroHPC JU Karolina supercomputer in the Czech Republic to address their project and successfully implement their observations.
The new data gathered will allow scholars to examine the causal effects of foreign aid on the likelihood of impoverished communities overcoming poverty. This, in turn, will enhance the alignment of development and aid initiatives with the challenges they aim to address.
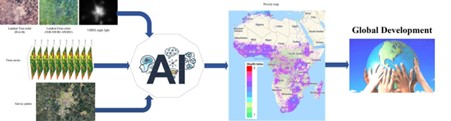
These two examples provide a small insight into the range innovation and discoveries that become attainable through the alliance of AI and HPC capabilities. When these two powerful technologies converge, new horizons in research open up, enabling us to delve deeper into complex problems, extract insights from vast datasets, and drive innovation across sectors.
by the EuroHPC JU
The EuroHPC JU will host a virtual workshop on AI on EuroHPC Supercomputers on 26 September 2023. More details here.
Details
- Publication date
- 12 September 2023
- Author
- European High-Performance Computing Joint Undertaking