Filter by
Awarded Projects (325)
RSS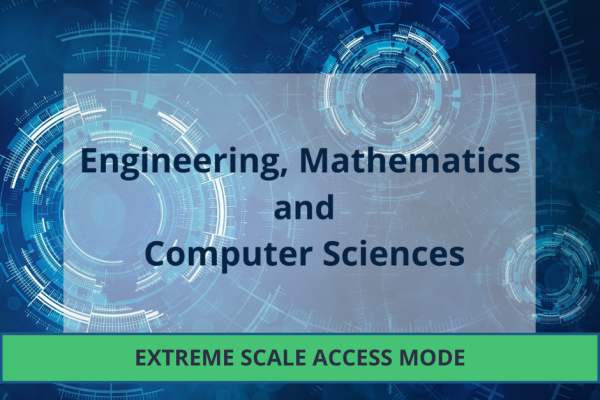
Among the solutions used to control shock wave/turbulent boundary layer interaction (SBLI) in practical applications, several studies in recent years have demonstrated the effectiveness of micro vortex generators (MVGs) to successfully delay shock-induced separation with reduced device drag.
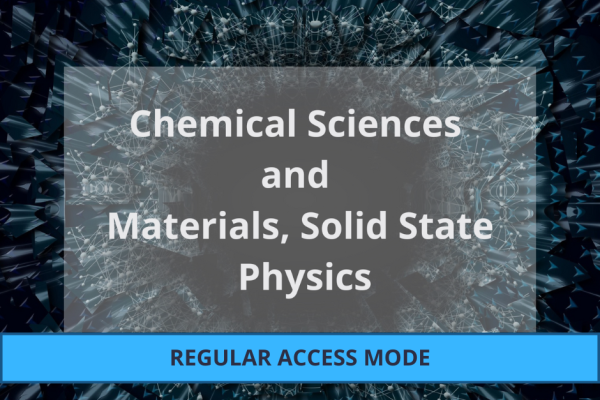
The design of novel photocatalytic and photovoltaic devices requires an in-depth understanding of the microscopic physical mechanisms governing the light-matter interaction.
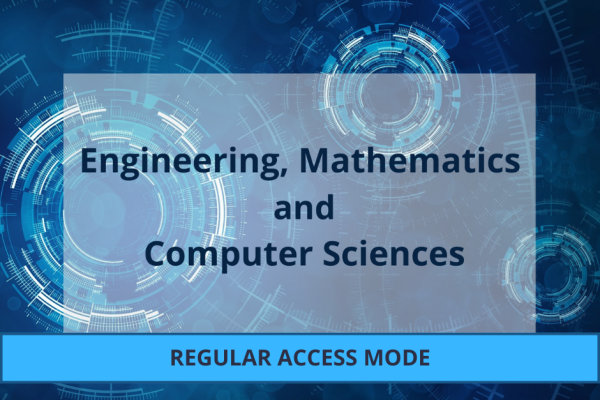
In this project, the research team proposes to leverage DNS to study a temporally-evolving nonequilibrium 3DTBL in a plane channel with an imposition of sudden transverse pressure gradient.
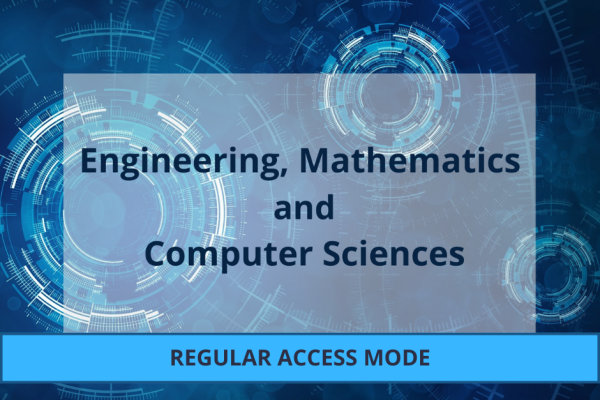
We investigate the effectiveness of wall-manipulation strategies based on the use of “spanwise traveling waves” in the high Reynolds number regime.
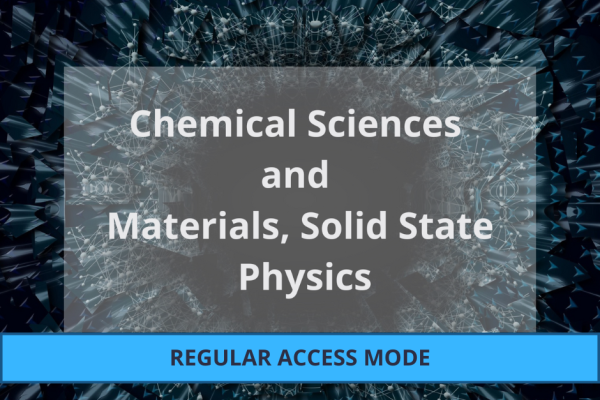
Addition of polymers to single-phase turbulent flows leads to a significant drag reduction. Based on the percentage of achieved drag reduction (DR) in the flow, the viscoelastic flows are categorized as low drag reduction (LDR) and high drag reduction (HDR) regimes.

The DYNAMITE (DYnamic biomolecular interaction Network And Machine learning Integration for Targeted Energy calculations) aims to revolutionize binding free energy (BFE) predictions by integrating large-scale molecular dynamics (MD) simulations with advanced machine learning (ML) methodologies.
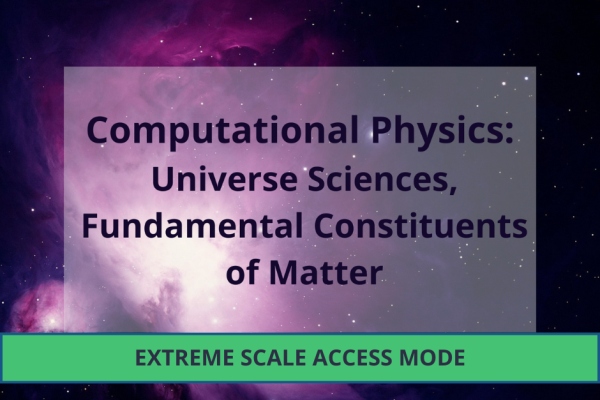
This project proposes to run global convection simulations with GPU-accelerated MHD solver (Pencil Code - Astaroth; PC-A) to study these processes in an unprecedented parameter regime.
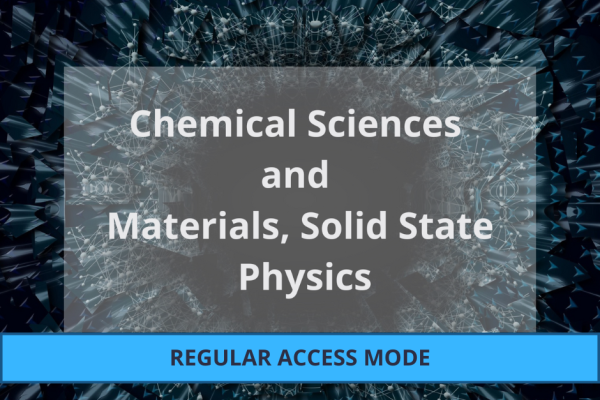
Phase-change processes are present in everyday life, nature, technology and in scientific applications. In particular, the melting of ice into salty water is directly linked to the melting of icebergs and glaciers into the ocean.
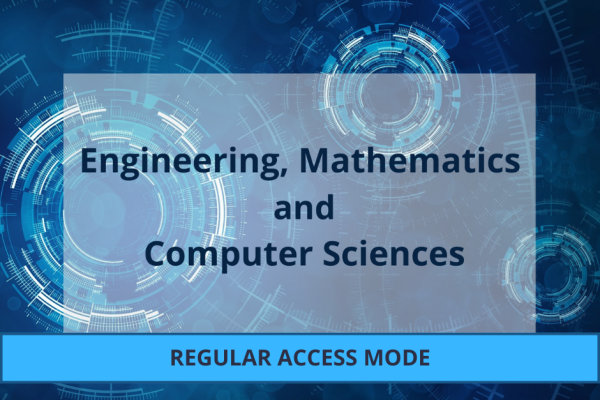
This proposed study aims to explore the effects of soluble surfactant contamination on heat transfer in a turbulent bubbly channel flow using our in-house high-fidelity front-tracking code.
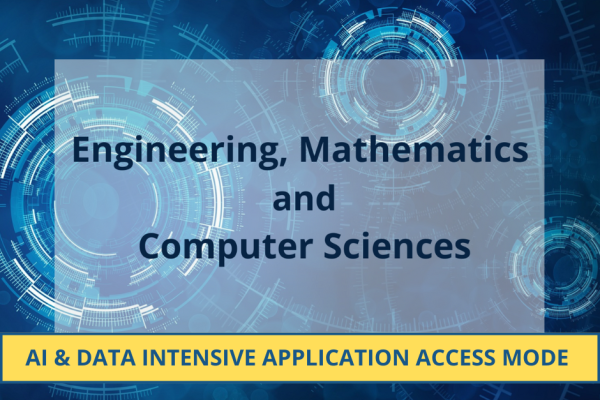
Inspired by dual-system cognitive theories, the project proposes a hybrid framework where a lightweight, fast-acting model operates in real-time while a larger, high-capacity model predicts future representations and refines decision-making.